Note
Click here to download the full example code
Point Interpolation¶
Compares different point interpolation approaches.
import cartopy.crs as ccrs
import cartopy.feature as cfeature
from matplotlib.colors import BoundaryNorm
import matplotlib.pyplot as plt
import numpy as np
from metpy.cbook import get_test_data
from metpy.interpolate import (interpolate_to_grid, remove_nan_observations,
remove_repeat_coordinates)
from metpy.plots import add_metpy_logo
def basic_map(proj, title):
"""Make our basic default map for plotting"""
fig = plt.figure(figsize=(15, 10))
add_metpy_logo(fig, 0, 80, size='large')
view = fig.add_axes([0, 0, 1, 1], projection=proj)
view.set_title(title)
view.set_extent([-120, -70, 20, 50])
view.add_feature(cfeature.STATES.with_scale('50m'))
view.add_feature(cfeature.OCEAN)
view.add_feature(cfeature.COASTLINE)
view.add_feature(cfeature.BORDERS, linestyle=':')
return fig, view
def station_test_data(variable_names, proj_from=None, proj_to=None):
with get_test_data('station_data.txt') as f:
all_data = np.loadtxt(f, skiprows=1, delimiter=',',
usecols=(1, 2, 3, 4, 5, 6, 7, 17, 18, 19),
dtype=np.dtype([('stid', '3S'), ('lat', 'f'), ('lon', 'f'),
('slp', 'f'), ('air_temperature', 'f'),
('cloud_fraction', 'f'), ('dewpoint', 'f'),
('weather', '16S'),
('wind_dir', 'f'), ('wind_speed', 'f')]))
all_stids = [s.decode('ascii') for s in all_data['stid']]
data = np.concatenate([all_data[all_stids.index(site)].reshape(1, ) for site in all_stids])
value = data[variable_names]
lon = data['lon']
lat = data['lat']
if proj_from is not None and proj_to is not None:
try:
proj_points = proj_to.transform_points(proj_from, lon, lat)
return proj_points[:, 0], proj_points[:, 1], value
except Exception as e:
print(e)
return None
return lon, lat, value
from_proj = ccrs.Geodetic()
to_proj = ccrs.AlbersEqualArea(central_longitude=-97.0000, central_latitude=38.0000)
levels = list(range(-20, 20, 1))
cmap = plt.get_cmap('magma')
norm = BoundaryNorm(levels, ncolors=cmap.N, clip=True)
x, y, temp = station_test_data('air_temperature', from_proj, to_proj)
x, y, temp = remove_nan_observations(x, y, temp)
x, y, temp = remove_repeat_coordinates(x, y, temp)
Natural neighbor interpolation (MetPy implementation)¶
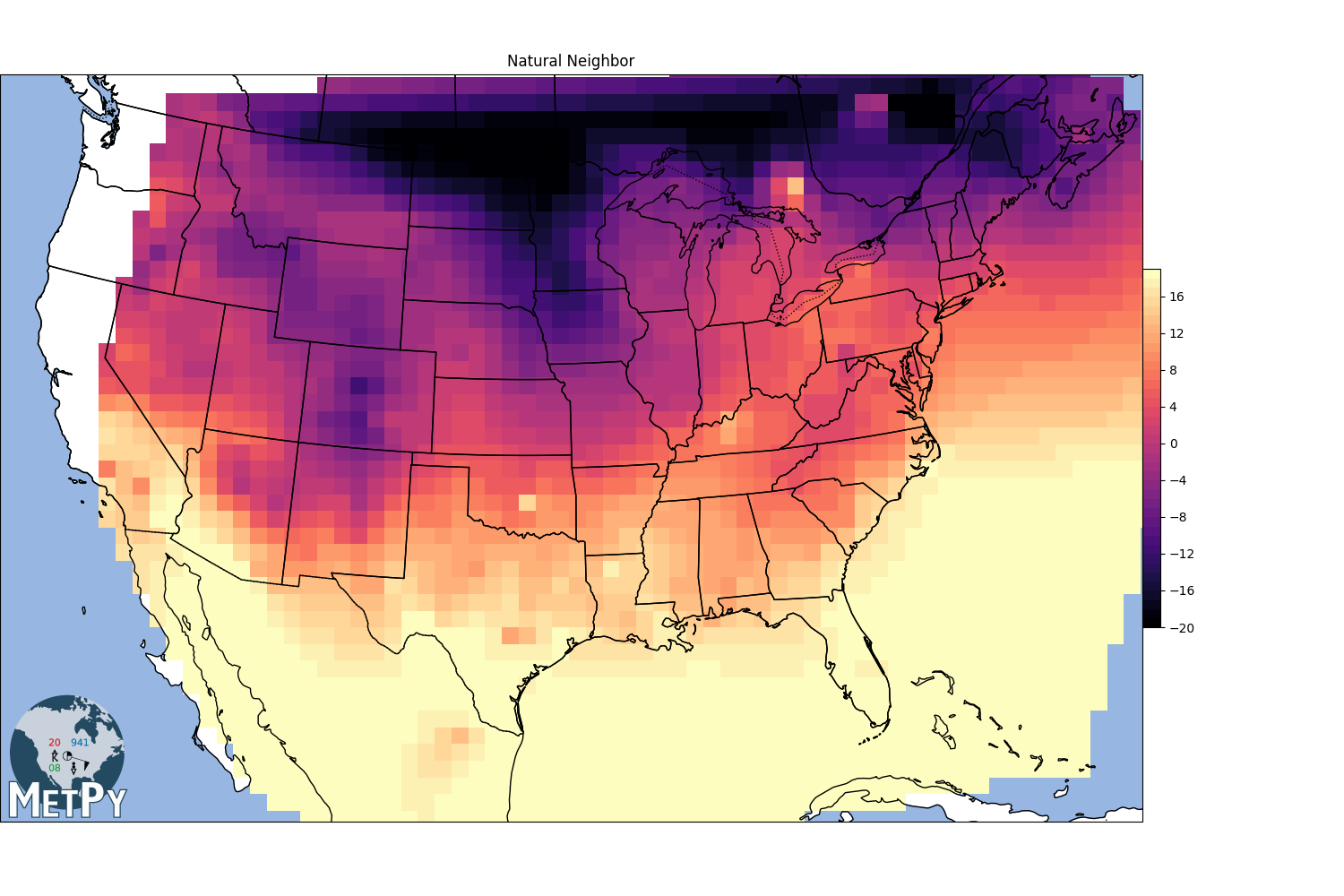
Out:
<matplotlib.colorbar.Colorbar object at 0x7fef2c05d0d0>
Cressman interpolation¶
search_radius = 100 km
grid resolution = 25 km
min_neighbors = 1
gx, gy, img = interpolate_to_grid(x, y, temp, interp_type='cressman', minimum_neighbors=1,
hres=75000, search_radius=100000)
img = np.ma.masked_where(np.isnan(img), img)
fig, view = basic_map(to_proj, 'Cressman')
mmb = view.pcolormesh(gx, gy, img, cmap=cmap, norm=norm)
fig.colorbar(mmb, shrink=.4, pad=0, boundaries=levels)
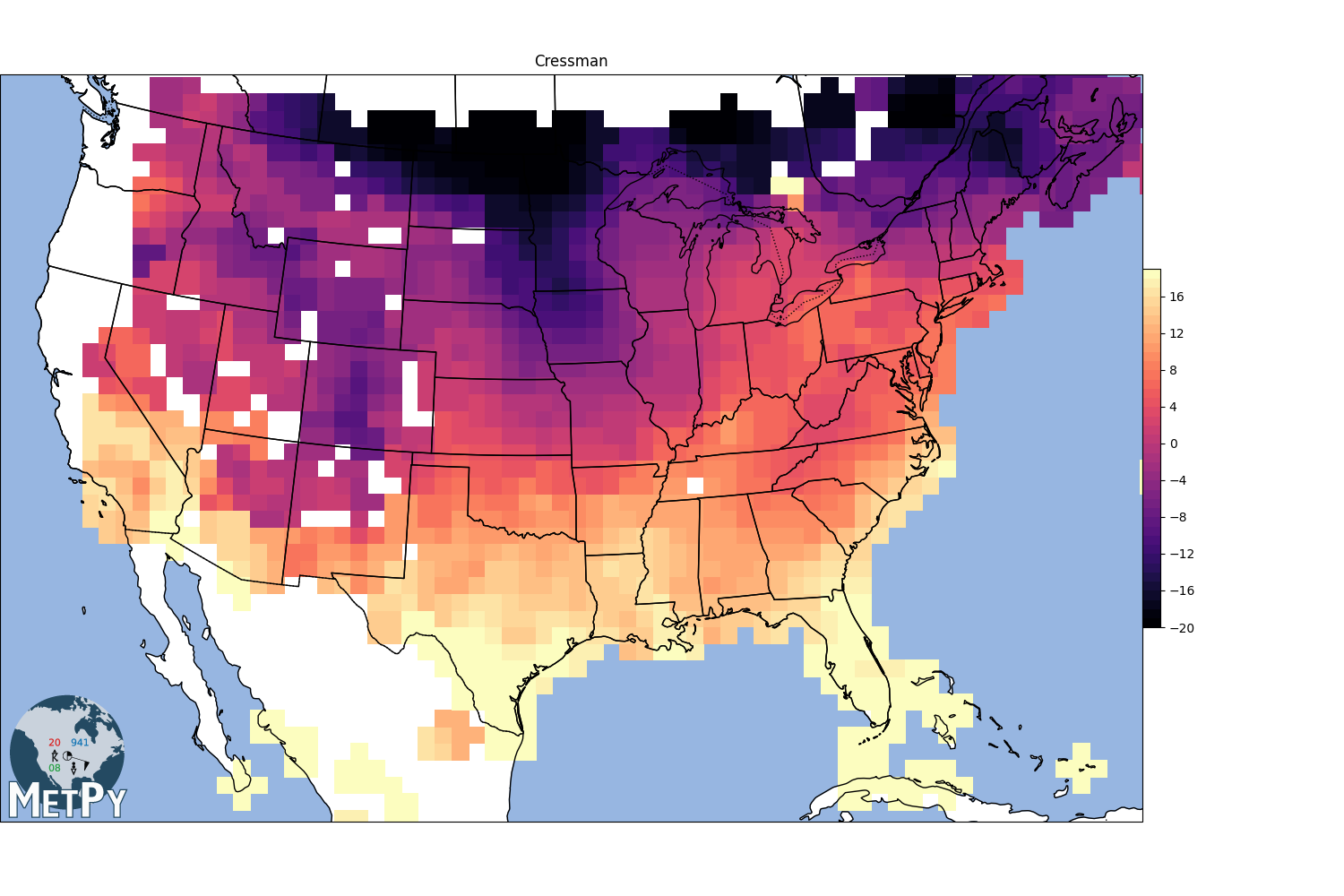
Out:
<matplotlib.colorbar.Colorbar object at 0x7fef05da2d60>
Barnes Interpolation¶
search_radius = 100km
min_neighbors = 3
gx, gy, img1 = interpolate_to_grid(x, y, temp, interp_type='barnes', hres=75000,
search_radius=100000)
img1 = np.ma.masked_where(np.isnan(img1), img1)
fig, view = basic_map(to_proj, 'Barnes')
mmb = view.pcolormesh(gx, gy, img1, cmap=cmap, norm=norm)
fig.colorbar(mmb, shrink=.4, pad=0, boundaries=levels)
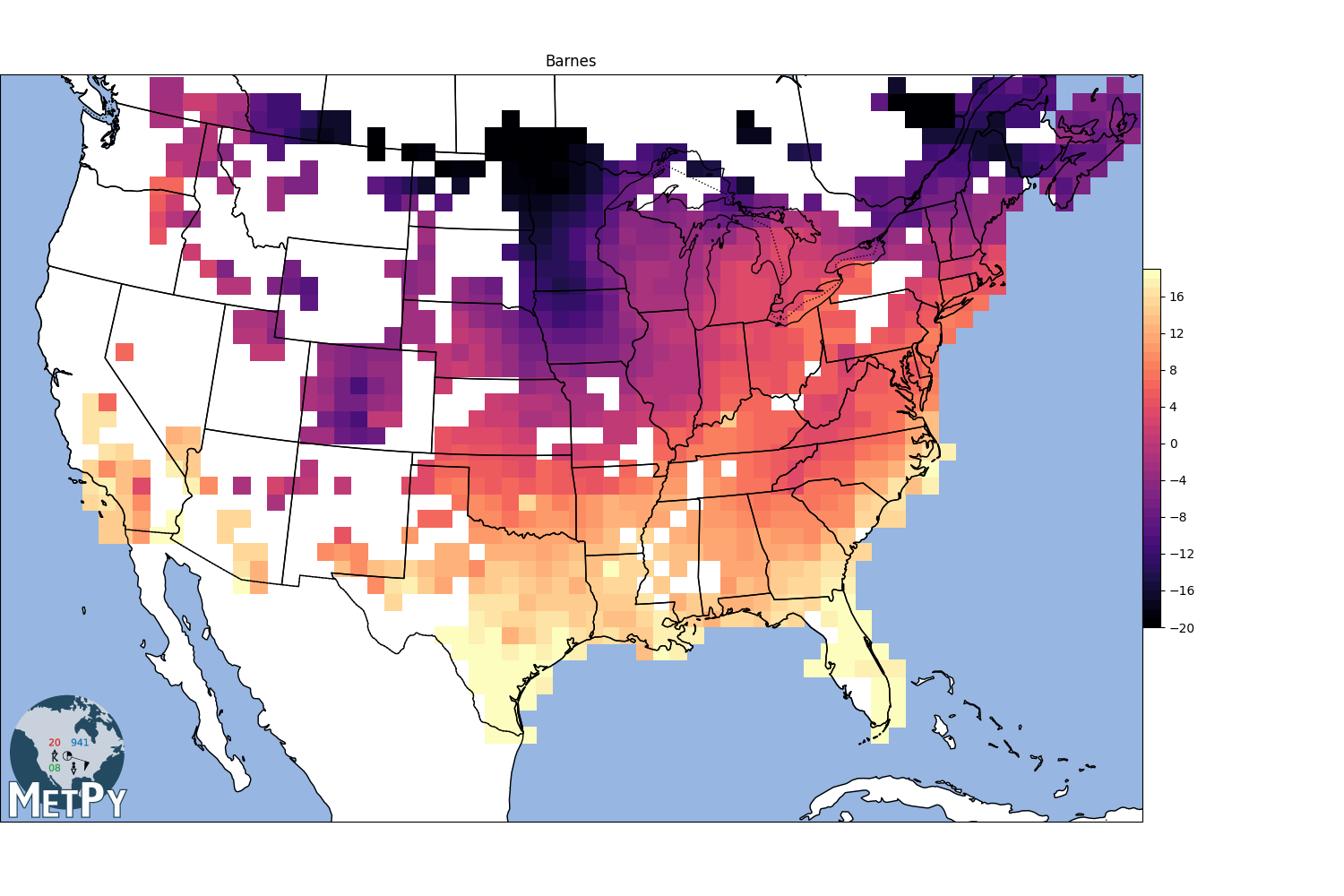
Out:
<matplotlib.colorbar.Colorbar object at 0x7fef2c2461f0>
Radial basis function interpolation¶
linear
gx, gy, img = interpolate_to_grid(x, y, temp, interp_type='rbf', hres=75000, rbf_func='linear',
rbf_smooth=0)
img = np.ma.masked_where(np.isnan(img), img)
fig, view = basic_map(to_proj, 'Radial Basis Function')
mmb = view.pcolormesh(gx, gy, img, cmap=cmap, norm=norm)
fig.colorbar(mmb, shrink=.4, pad=0, boundaries=levels)
plt.show()
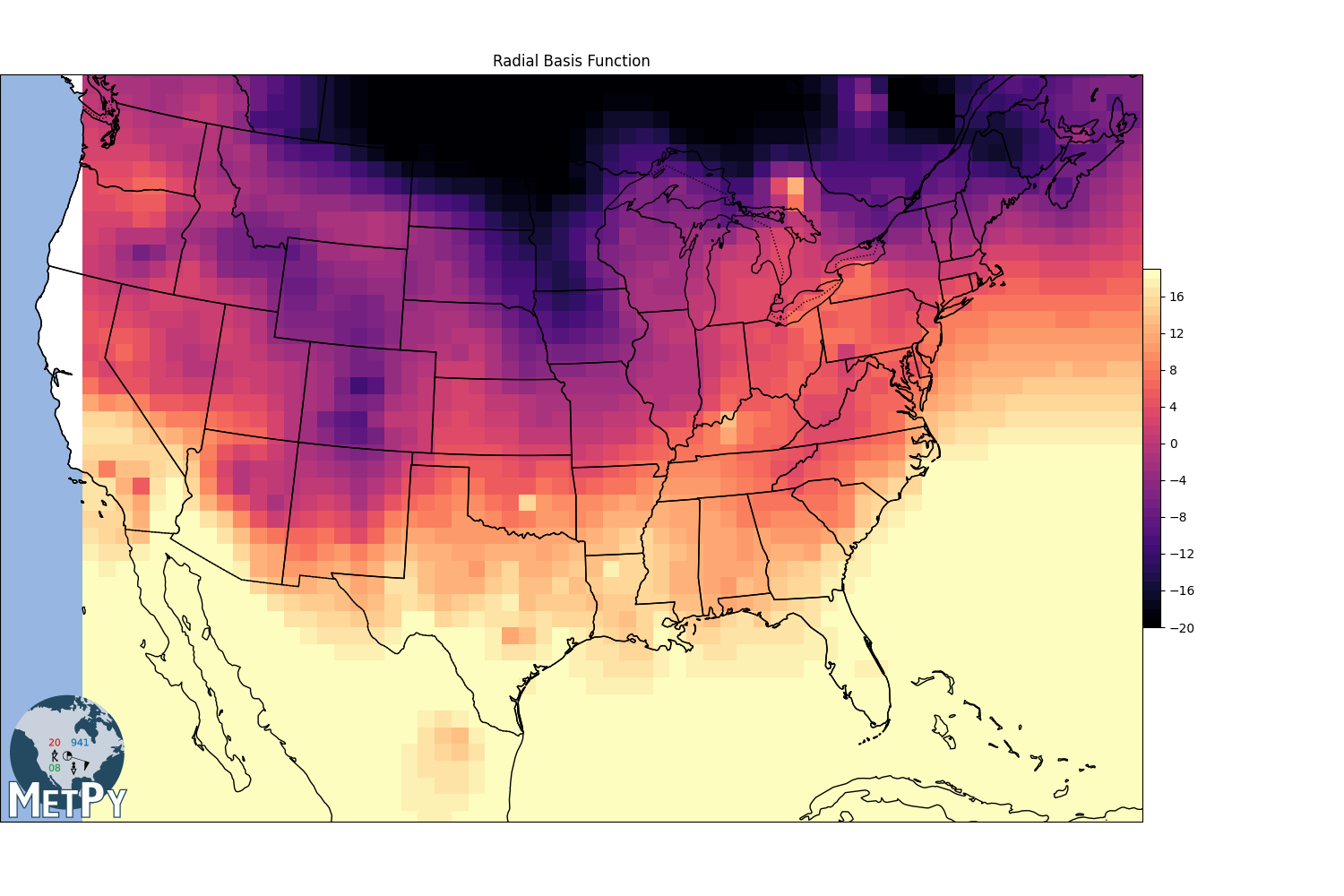
Total running time of the script: ( 0 minutes 6.987 seconds)